本论文介绍我们获得国家发明专利及美国专利授权的一种关于全方向自适应柔性多面体网络结构的视触融合本体感知学习技术,可以在实现物品形貌全方向自适应形变的同时,通过我们提出的指内视觉感知方案以及柔性超材料动态粘弹性建模,结合机器人学习方法,实现高性能六维力与力矩的精细检测以及实时本体形变三维重建,可用于柔性力触觉感知抓取、接触物品形貌重建等场景,同时具有极低的成本优势、且能在百万次循环下仍能保持良好的力触觉感知性能,在相关领域具有较好的技术前沿优势与水陆两栖应用前景。该论文发表于《The International Journal of Robotics Research》,共同第一作者包括南方科技大学博士研究生刘小博和韩旭东,合作作者包括南方科技大学力航系教授洪伟,通讯作者为设计学院助理教授万芳、机械与能源工程系助理教授宋超阳
The Soft Polyhedral Network with an embedded vision for physical interactions offers a low-cost, lightweight, and adaptive solution for robotic proprioception, capable of learning kinetic features for accurate real-time force and torque detection, and demonstrating its versatility in tasks like sensitive grasping and touch-based geometry reconstruction through over one million use cycles.
DOI: https://doi.org/10.1177/02783649241238765
2024年3月13日,南方科技大学团队在国际学术期刊The International Journal of Robotics Research上,以“Proprioceptive Learning with Soft Polyhedral Networks”为题在线发表研究论文,系统介绍了一类可实现全方向自适应交互的柔性多面体网络结构设计方法,率先提出基于指内视觉的机器人本体感知学习技术,揭示了柔性超材料中动态粘弹性力学对于大形变下精细力触觉感知学习的影响机制,同时实现了柔性力觉精细检测以及实时动觉三维重建,可用于机器人力触觉感知抓取、柔性智能人机交互、接触物品形貌重建等应用场景,且在百万次循环使用下仍能保持可靠的视触融合本体感知性能。该论文的共同第一作者为2019级博士研究生刘小博和2021级博士研究生韩旭东,合作作者为南方科技大学力航系教授洪伟,通讯作者为创新创意设计学院助理教授万芳、机械与能源工程系助理教授宋超阳。
In robotics, one of the enduring challenges has been to replicate the dexterity and adaptability of human fingers. Our fingers possess a unique neuromuscular sense known as proprioception, which allows us to precisely handle objects by sensing position, movement, force, and effort. While considerable research has been into artificial skins and robotic end-effectors, achieving seamless integration of these components into a coherent robotic system has proven to be complex.
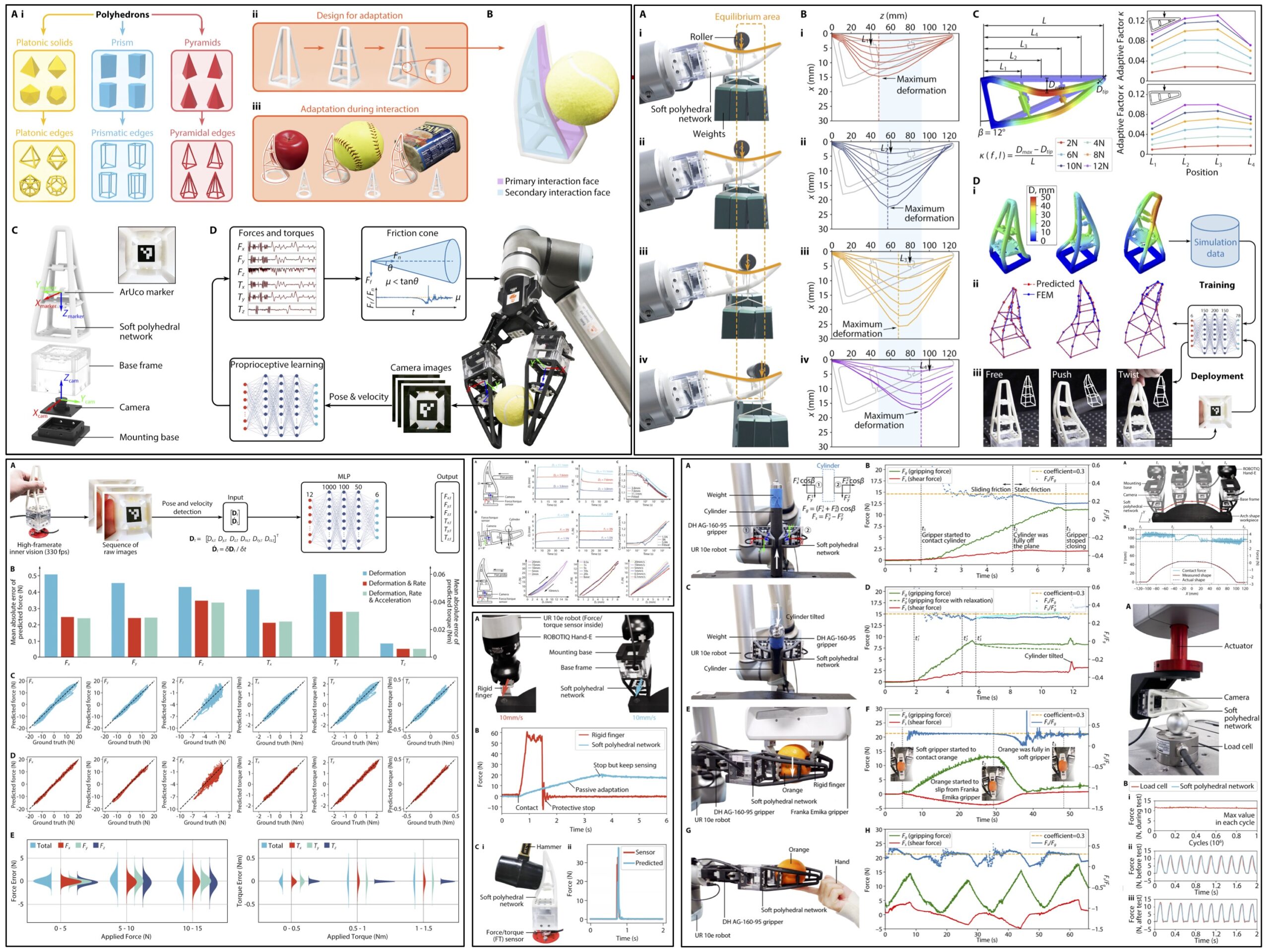
本体感知,也就是人们常说的“第六感”,是一种通过运动神经元帮助我们实现对身体四肢空间姿态的感受与认知的能力,包括对关节位置与运动的动觉感知、以及对肌肉力量与程度的力觉感知,需要借助肌肉骨骼系统与多种感受器官之间的相互配合得以实现。如何实现机器智能的“第六感”技术,是研制新一代智能机器人技术的一项重要挑战,需要模仿人类手指在抓取物品时的自适应性包覆、关节位置感知、以及接触过程中的精细力触觉感知等多方面性能,在实现对物品形貌精细重建的同时还需要在实际应用中以更低的成本提供简单可靠且柔性智能的交互方式,目前仍然是智能机器人技术领域的一大难题。
Our research addresses this challenge by developing Soft Polyhedral Networks, a design that enables vision-based proprioception with passive adaptations in all directions. This work builds upon our previous research in vision-based tactile sensing, expanding its capabilities to include mechanically programmable adaptation under passive interaction. By transforming any polyhedral geometry into a soft network, we have created a system that can be integrated into robotic fingers, enhancing their ability to interact with the physical world and human operators.
在本项目的前期研究中,南科大团队自主独立发明了一种通用设计方法,可实现具有全方向大形变自适应性能的柔性多面体网络结构(Soft Polyhedral Network)设计,该结构在非结构化物理交互过程中可以提供成本低廉、性能优越、水陆通用、稳定可靠的全方向自适应包覆性能,并已经获得了多项国家发明专利及美国专利授权。在此基础上,南科大研究团队进一步提出基于指内视觉的柔性交互微型运动捕捉系统设计,实现对柔性网络结构整体大形变过程的高帧率无遮挡全方向运动捕捉,基于大规模有限元仿真数据的系统采集与实验标定,实现了整个柔性多面体网络结构实时形变的仿真向真实(Sim2Real)学习,实现了对该柔性多面体网络结构关节位置和运动的高性能实时动觉感知。
A key aspect of our design is adding a miniature motion-tracking system at the base of the robotic finger. This system allows us to capture and encode the soft network’s whole-body deformation in real time by tracking the spatial movement of a fiducial marker placed inside. This capability enables us to study the viscoelasticity of the soft metamaterial in detail, an essential factor often overlooked in soft robotics.
在此基础上,南科大研究团队进一步针对非结构交互过程中由于物理接触引起的力觉感知,进行了深入研究。在本研究中提出了基于指内视觉的柔性精细力触觉感知与机器人学习技术,深入研究了这一类柔性超材料网络结构的动态粘弹性机理,采用视触融合的方法进行了精细的力触觉表征与自适应交互性能实验,验证了该方案在百万次循环条件下仍具有良好的力触觉感知性能,并通过对柔性交互速度项的引入,显著提升了对柔性力触觉六维交互力与力矩的预测精度并达到国际领先水平,可基于此进一步实现对包括接触摩擦力等多项力觉感知与交互性能参数的精确估计。
To accurately model the viscoelasticity of the soft network for dynamic proprioception, we have developed a data-driven model that incorporates both deformation and kinetic input features. This approach has led to state-of-the-art force sensing, achieving accuracies of 0.25/0.24/0.35 N for force and 0.025/0.034/0.006 Nm for torque. The soft networks can be attached to almost any rigid or soft grippers of compatible sizes, providing high-performing proprioception and omni-directional adaptation at low costs in mechanical design and algorithmic computation.
通过上述的柔性动觉与精细力觉的深入研究,南科大团队系统提出基于柔性多面体网络结构的本体感知学习技术,实现了机器人指末端的“第六感“。在本研究中,南科大团队通过多方面的系统实验,展现了采用指内视觉的柔性多面体网络结构在兼备成本低廉、性能优越、水陆通用、稳定可靠等硬件优势同时,借助视触融合精细力感知技术,可以胜任多种极具挑战的非结构场景下的人机交互任务,包括对接触摩擦力的精准预测、物品几何形貌的大形变力触觉重建、相比刚性指末端更加优越的力触觉感知与无损抓取性能、以及可以与人类手指相接近的自适应力触觉交互性能。结合该专利授权设计方法的通用性,未来可拓展品类丰富、应用广泛、平价可靠、力触感知的人机交互场景,特别在诸如面向具身智能的多模态力触觉感知与学习、水下极端环境下的精细力触觉物品操控等不同生活、工业、及特种场景,具有良好的科学理论基础与广阔的技术应用潜力。
该研究得到了国家自然科学基金青年基金项目、国家自然科学基金重点项目、深圳市基础研究专项重点项目、深圳市智能机器人与柔性制造重点实验室、广东省人体增强与康复机器人重点实验室的资助。
In conclusion, our research on Soft Polyhedral Networks represents a significant step forward in enhancing robotic systems’ proprioceptive abilities. By combining vision-based sensing with soft robotics, we have developed an adaptable, accurate, and durable design, opening up new possibilities for robotic interaction with the physical world and human operators.
Xiaobo Liu#, Xudong Han#, Wei Hong, Fang Wan*, and Chaoyang Song* (2024). “Proprioceptive Learning with Soft Polyhedral Networks.” The International Journal of Robotics Research, 0(0):1-20, (OnlineFirst).
doi: https://doi.org/10.1177/02783649241238765